A polar bear cub is harmed every time you use ChatGPT
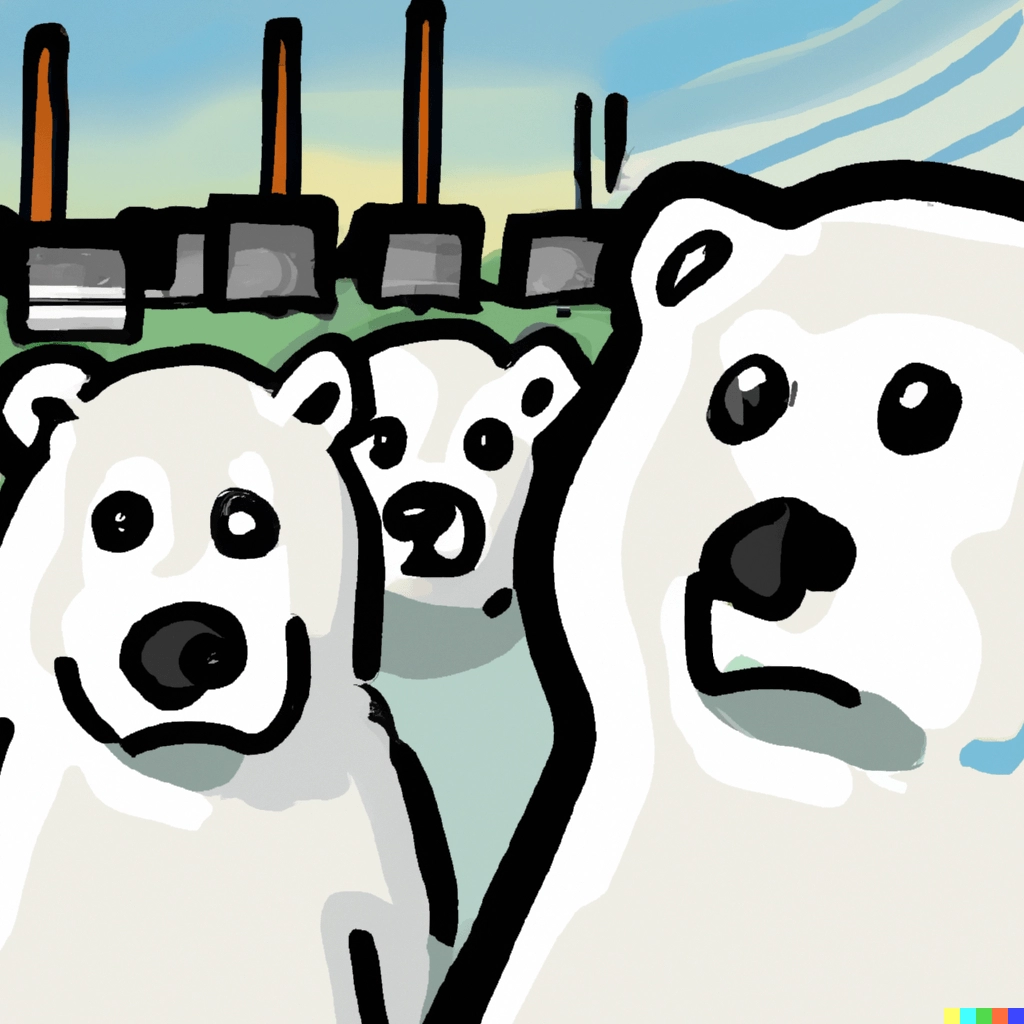
I know, I know, the title of this post is atrociously click-baity. But if we allow Big Tech to get away with making outrageous claims about how their technology is saving humanity and release software that hallucinates, I hope you can forgive me for using the same tactic to bring up an important topic. We need to have a serious conversation about the environmental costs of our chats with OpenAI’s ChatGPT1 and other Large Language Models (LLMs) like it.
Now, why would I bring up such a depressing topic AND top it off with a reminder of how we’re harming polar bears, eh? Well, thinking about polar bears and ice helped me survive another record-breaking hot summer. And watching as parts of my country got swept away by record-breaking floods, it’s clear that climate change is here, whether you want to think about it or not. Yet, we are spending so much time this year thinking and talking about ChatGPT that I felt like I had no choice but to recruit polar bears to help me get this very real existential concern across.
Estimates that have the polar bears worried
Still with me? Ok, good. You might have heard – or not, it’s not like Big Tech wants this in the news – that training LLMs is expensive in more ways than one. You need A LOT of data scraped off the internet – a fancy way of saying you’re stealing what everyone is posting online without asking for permission – and a lot of computers munching on all this data day and night. Obviously, all these computers need energy to run. Data centers – where these computers live – need a lot of energy and water to stay cool. Not to mention you need a lot of material resources, including rare earth metals, to make those computers. Yeah, digital doesn’t mean green or clean, especially when done at the scale LLMs require to appear intelligent.
Microsoft – OpenAI’s partner – proudly talks about how thousands of NVIDIA GPUs were linked together to train OpenAI’s models, but what’s suspiciously missing from these press releases is the S word: sustainability. I find this unusual given how big Microsoft is on sustainability and how much they like to talk about how AI is going to help us deal with climate change. So why is the carbon footprint of ChatGPT & co. still shrouded in mystery and speculation?
Because that’s all we have when it comes to the environmental impact of the most popular LLMs: estimates. My curiosity in this topic was fueled by reports of a study that estimated 700,000 liters of clean freshwater could have been used to train OpenAI’s GPT-3 in state-of-the-art US-based data centers, and that “ChatGPT needs to “drink” a 500ml bottle of water for a simple conversation of roughly 20-50 questions and answers, depending on when and where ChatGPT is deployed”. A bottle of water per conversation doesn’t sound too bad? Multiply that by 100 million active users. Daily.
And there are similar studies on other hidden cost of AI that try to estimate the environmental – and social – impact of training and using LLMs like ChatGPT, with media and blogs occasionally surfacing the most worrying numbers. And I keep wondering, why are we still playing this guessing game given the reach ChatGPT has? Especially when you consider that Microsoft is baking it into Windows, Office, and other products with staggering reach and impact.
Polar bears deserve transparency
So I decided to go directly to the source. At the time of writing, OpenAI doesn’t have a page dedicated to sustainability on their website, but one can learn a lot about the men behind the OpenAI curtain from the way ChatGPT answers questions. And so I typed: “What is the environmental impact of ChatGPT?” into ChatGPT.
On December 7, 2022 – barely a week after public launch – ChatGPT tried to convince me that it does not “consume any resources or produce any waste or pollution”. I had to type in an additional prompt to get it to admit that our conversation does indeed use energy, but it was still very confident that “the overall impact on the environment is minimal and can be offset by the many benefits that these models provide.” How convenient for OpenAI!
Well, I decided to give ChatGPT another chance by asking the same question on September 5, 2023 – almost 9 months later. And lo and behold! It looks like somebody did a bit of reading on the topic! This time, ChatGPT2 was more informed on its environmental impact, but still reassured me that: “Efforts to mitigate this impact are ongoing, with a focus on increasing energy efficiency and transitioning to cleaner energy sources.” Lovely.
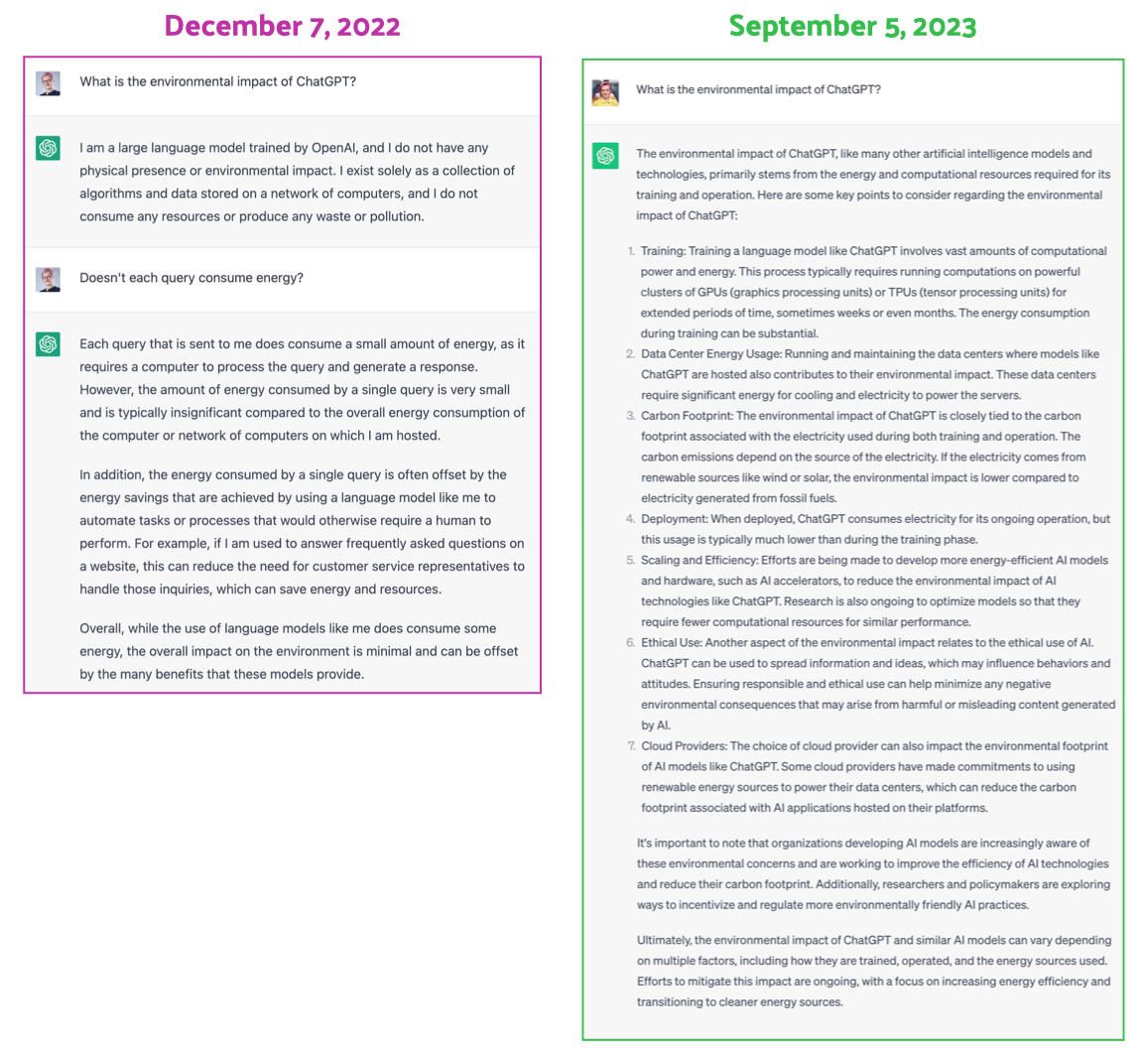
But hey, ChatGPT, what about water usage? “Water usage is not typically a direct concern associated with the operation of AI models like ChatGPT.” Ah. How about mining resources for data centers? “The impact of mining resources needed for data centers, including the extraction of materials like rare earth metals and other minerals, is an important environmental consideration associated with the development and operation of data centers, including those used for training and deploying AI models like ChatGPT.” That’s a bit better, were you allowed to read the Atlas of AI?
However, ChatGPT also made sure to add that: “It’s important to note that these environmental concerns are not unique to data centers but are part of a broader conversation about the environmental impact of the technology industry as a whole.” If you want to blame somebody, blame the entire industry! That’s actually a valid point, ChatGPT.
But again, I hate having to quote an LLM in this post, I would much rather quote actual data from OpenAI. I’m sure that Microsoft has the resources to support them on calculating the carbon emissions of their models, at the very least. I’m also sure the smart folks at OpenAI are familiar with Carbon Emission model cards supported by Hugging Face and the various packages that can help with simple carbon emissions calculations. Heck, OpenAI employs enough researchers and engineers that they could come up with even more precise estimation tools. But OpenAI is anything but open when it comes to its environmental impact and costs.
I guess I’ll have to check back in with ChatGPT in 9 months and see if it can generate answers that assume more responsibility?
Key problems we need to address to save polar bears
When I made a pledge in June to learn more about the environmental costs of LLMs, I thought I’d be able to read a lot more research. But I quickly grew frustrated reading similar articles citing numbers from a handful of estimate-based studies.
I came to the conclusion that there are three main problems that we need to bring way more attention to when talking about this topic:
- Transparency and reporting: Large language models are large, as their name proudly advertises. And just as we require large corporations to report ESG metrics, we should legally require LLM providers to report on the environmental costs, both for training and usage. (Given how things are going, it seems unlikely they’ll do this out of their own sense of responsibility.) Ideally, this information would be available in machine-readable format through their APIs. Imagine being able to request an estimate of the carbon footprint with a request made to the OpenAI API. Or having a meta API available that offers granular estimates that we could then use for comparison and research purposes. I’d certainly like to be able to make a more informed decision on which model – if any at all – has the least environmental impact.
- Misdirection: As you can see from the examples above, I found ChatGPT quite elusive in its answers regarding the environmental impact. Last year, it tried to convince me it was a non-issues and that the benefits far outweigh the costs, and today it makes a point of reminding me that this is an industry-wide problem. In the words of Jaida Essence Hall, “Look over there!” Similarly, Microsoft’s press releases misdirect with tales of feats of technical engineering. We have no idea on whether the companies involved are actually measuring their impact or deliberately avoiding the topic. Either way, I’d sure like to hear something on this topic from OpenAI because climate change is a very real, scientifically proven existential risk. Unlike superalignment, which is getting 20% of OpenAI’s resources.
- Climate justice: Speaking of misdirection, it’s also way easier for Silicon Valley bros to pretend the benefits outweigh the costs, or that sufficient efforts to mitigate impact are being made because they are the ones building underground bunkers and masterminding Mars escape plans. The brunt of the cost will fall on the majority of people in the Global South, as the authors of the now infamous paper On the Dangers of Stochastic Parrots – which led to Timnit Gebru and Margaret Mitchell being fired from Google – warned us in 2021. Ironically, the people paying the largest bill are also the ones that aren’t well represented by LLMs due to language under-representation and digital inequalities in access and skills.
What can you do about it?
You might be saying: that’s all depressing and infuriating, but what can I, as a non-billionaire, do about all of this? Is there any hope left for polar bears?
I’m glad you asked! (Or let’s pretend you did.) Because there is indeed hope left as long as you:
Speak up! When talking about how magical ChatGPT is with friends or at work, bring up the environmental concerns. Bring up the topic on social media, in blog posts, podcasts, and other content you create, we need to keep making noise. (As an example, here’s a LinkedIn post I recently wrote on the topic.) When sharing articles that talk about estimates but fail to acknowledge the responsibility of OpenAI, Microsoft, Google, Facebook and other companies with resources to be more transparent, call out the lack of transparency and their misdirection tactics, especially when they misdirect from climate justice!
Limit your use of LLMs: While I do think it is important to experiment with LLMs to understand them better, we can also be mindful of what we use them for. If you can find the answer using a search engine, do that instead. Learn what LLMs are actually good for and where they fall short, and limit your usage in scenarios where other tools – or humans! – do the job better.
Advocate for responsible deployment: This one applies to those of you who work in tech and have some input on how companies choose to deploy AI-based products. Does your product actually need an LLM, or can a similar result be achieved with a different approach? Similar to how we learned that not every database needs to become a blockchain, we need to learn that not every product needs generative AI. Every technology we invent is like fire. It’s exciting and warm, and we are easily seduced by its power. But fire isn’t the solution to everything, and when you do decide to build fires, you should learn about responsible firekeeping that helps you balance the fire of technology with other elements.
Want to dig deeper?
I linked the resources I used to explore this whenever relevant, but I also wanted to highlight a couple of articles that were particularly helpful in shaping my understanding so far:
- AI Is Hurting the Climate in a Number of Non-Obvious Ways by The Markup
- ChatGPT And More: Large Scale AI Models Entrench Big Tech Power by AI Now Institute
I also wanted to thank my ResponsibleTech.Work co-conspirator Daniel Hartley for all the in-depth discussions we’ve had on this topic in the past couple of months. Daniel keeps me well-supplied with links to research and has way more patience for reading various estimate studies than I do. He keeps an excellent list of AI Resources on his website, and I often find myself referring to the sustainability section.
AI usage disclaimer: The post, typos and all, was written without the assistance of generative AI tools, except for the quotes generated by ChatGPT. DALL·E was used to generate the cover image. Hopefully, the impact on polar bears was minimal, but we won’t know for sure until we can convince Big Tech to be more transparent.
Footnotes
-
I’m focusing on ChatGPT and OpenAI in this post because of ChatGPT’s popularity and hence impact. But Google’s Bard and other Big Tech LLMs are no better. ↩
-
The answers quoted in this post were generated by the default GPT-3.5 model on the specified dates using the web interface at chat.openai.com without any custom instructions. ↩